João Pedro Quintais
Klewer Copyright Blog, Link
Generative AI is one of the hot topics in copyright law today. In the EU, a crucial legal issue is whether using in-copyright works to train generative AI models is copyright infringement or falls under existing text and data mining (TDM) exceptions in the Copyright in Digital Single Market (CDSM) Directive. In particular, Article 4 CDSM Directive contains a so-called “commercial” TDM exception, which provides an “opt-out” mechanism for rights holders. This opt-out can be exercised for instance via technological tools but relies significantly on the public availability of training datasets. This has led to increasing calls for transparency requirements. In response to these calls, the European Parliament is considering adding to its compromise version of the AI Act two specific obligations with copyright implications on providers of generative AI models: on (1) transparency and disclosure; and (2) on safeguards for AI-generated content moderation. There is room for improvement on both.
- As currently worded, the transparency obligation to “document and make publicly available a summary of the use of training data protected under copyright law” is impossible to comply with. As such, it is necessary to reconsider this provision in light of the intended policy aim, including focusing on access to datasets, incentivizing cooperation with rights holders, and possibly standardization of opt-outs.
- The safeguards provision should learn from recent policy discussions on permissible filtering of copyrighted material and clarify the need for measures deployed by providers of generative AI to ensure the protection of transformative uses based on freedom of expression.
Introduction
Generative AI is one of the hot topics in copyright law today. By now, you might have heard of some of the lawsuits filed against AI companies, alleging that they infringed on copyright in the training of their models. AI-generated vocals are roiling the music industry, with platforms acting to take down infringing content. Authors and copyright holders are concerned that generative AI tools are built on the unauthorized and unremunerated use of their works, while at the same time negatively impacting their livelihood. As a counterpoint, some commentators note that these tools benefit many artists and content creators, whose interests should be considered when regulating how copyright law tackles these technologies. Others still are concerned that legal intervention at this stage would lead to market concentration and “make our creative world even more homogenous and sanitized”.
The biggest copyright law question in the EU and US is probably whether using in-copyright works to train generative AI models is copyright infringement or falls under the transient and temporary copying and TDM exceptions (in the EU) or fair use (in the US). In the EU, the emergence of generative AI has disrupted the legislative process for the proposed AI Act and forced lawmakers to reconsider how they categorize and assign responsibilities to providers and users of AI systems (see here). Although the AI Act is not specifically intended for copyright law, EU lawmakers are currently considering requiring providers of generative AI systems that they “make publicly available a summary disclosing the use of training data protected under copyright law.”
This post addresses this issue by placing it in the broader context of how EU copyright law tackles generative AI, examining how the proposed AI Act provisions interface with EU copyright law, and reflecting on its potential benefits and risks as regards transparency of data sets and moderation of AI generated content.
Inputs and Outputs: How to approach copyright law issues with generative AI
[N.B. Readers familiar with these issues should jump directly to the next section]
One way to consider the copyright aspects of generative AI tools is to divide them into legal questions that deal with the input or training side vs. questions that deal with the output side.
From the input perspective, the main issue relates to the activities needed to build an AI system. In particular, the training stage of the AI tools requires the scrapping and extraction of relevant information from underlying datasets, which often contain copyright protected works. In the EU, these activities are mostly regulated by two text and data mining (TDM) exceptions in the 2019 Copyright in the Digital Single Market (CDSM) Directive, which cover TDM for scientific purposes (Article 3) and what is sometimes called “commercial” TDM (Article 4). For models like Midjourney, Dalle-E, or Firefly, the relevant provision would be the commercial TDM exception. The training activities of Stable Diffusion are trickier to qualify, since the company apparently paid a German non-profit, LAION, to inter alia produce a training dataset (LAION-5B) for its generative AI tool. Still, given the tight requirements of the scientific TDM exception in Article 3 CDSM Directive, it is more likely than not that the TDM activities of Stable Diffusion would at least partly fall under Article 4 CDSM Directive. The analysis below will focus on the EU TDM exceptions, especially Article 4 CDSM Directive.
In the US, absent a specific TDM exception, the legal question is whether these activities qualify as fair use. In the aftermath of cases like Authors Guild v. HathiTrust and Authors Guild v. Google, it has been argued that the US doctrine of fair use allows for a significant range of TDM activities of in-copyright works (see e.g. the work of Sag, Samuelson, and Lemley and Casey; for a critical framing of questions of fair use in dataset creation, see Khan and Hanna). The result is that US copyright law is arguably one of the most permissive for TDM activities in the world, especially when compared to laws that rely on stricter exceptions and limitations, like the EU (see here). This would make the US an appealing jurisdiction for companies to develop generative AI tools (as noted here).
From the output perspective, a number of copyright questions are relevant. Is an output from a generative AI system protected by copyright? Does such an output infringe on a copyrighted work of a third party, especially those works “ingested” during the training stage of the AI system? Under US law, is the output a “derivative work” of the “ingested” copyrighted works? Do any copyright exceptions apply to outputs that might otherwise infringe copyright?
Some of these input and output questions are already being litigated in the US and the UK, most notably in a class action litigation against providers of Stable Diffusion (see complaint and motion to dismiss), as well as in lawsuits brought by Getty Images (reported here; see also this early analysis by Guadamuz).[1] For additional discussion on copyright protection of outputs in the EU and US, including the US Copyright Office Guidance on works containing material generated by AI, see e.g. this Primer and FAQ, other sources on this, or watch this excellent presentation by Professor Pamela Samuelson.
The legal regime for Inputs in EU Copyright Law
[N.B. Readers familiar with the EU legal rules should jump directly to the next section]
The EU TDM exceptions
The CDSM Directive defines TDM as “any automated analytical technique aimed at analyzing text and data in digital form in order to generate information which includes but is not limited to patterns, trends and correlations.” As it is easy to understand, such a broad definition will cover many of the training activities needed to develop an AI system, especially of the machine learning type, including generative AI systems.
Articles 3 and 4 CDSM Directive then contain two TDM-related mandatory exceptions. Article 3 provides an exception for acts of TDM for the purposes of scientific research – covering both natural and human sciences – by research organisations and cultural heritage institutions, regarding works/subject matter to which they have lawful access, and subject to a number of additional conditions.[2]
Article 4 sets forth an exception for reproductions and extractions of lawfully accessed works/subject matter for the purposes of TDM. This is meant to add legal certainty for those acts that may not meet the conditions of the temporary and transient copy exception in Article 5(1) InfoSoc Directive. The new exception is subject to reservation by rights holders, including through “machine-readable means in the case of content made publicly available online”, for instance through the use of metadata and terms and conditions of a website or a service. Such reservation shall not affect the application of the TDM exception for scientific purposes in Article 3. This possibility of reservation is usually called the “opt-out” provision, and I’ll return to it below.
There is already significant scholarship in the EU that takes a critical view of these exceptions (see e.g. here). As multiple critics have noted, both TDM exceptions are restrictive and may exclude many important applications in this domain, especially as regards the development of AI technologies (see e.g. the excellent work of Margoni and Kretschmer, Ducato and Strowel, Geiger and Rosati, as well as the European Copyright Society Opinion on the topic). However, the emergence of generative AI and its clash with the copyright world, together with a favorable political landscape and timing, appear to have given the commercial TDM exception a new wind as a viable scalable policy option to tackle generative AI.
The emergence of the commercial TDM opt-out as a policy option
As noted, the commercial TDM exception provides an opt-out mechanism for rights holders. This provision is already being used in practice by some creators, for instance through tools provided by spawning.ai, like the HaveIBeenTrained website, which “allows creators to opt out of the training dataset for one art-generating AI model, Stable Diffusion v3, due to be released in the coming months.” According to data provided by the company, by late April this year, more than 1 billion pieces of artwork had been removed from the Stable Diffusion training set using this tool (reported here).
There is disagreement among commentators whether this is a desirable development. On the one hand, commentators like Paul Keller consider that this approach has the potential to increase the bargaining power of rights holders and lead to licensing deals with (and remuneration from) AI providers. Similarly, a recent Communia policy paper argues that the opt-out approach “constitutes a forward-looking framework for dealing with the issues raised by the mass scale use of copyrighted works for ML training […] it ensures a fair balance between the interests of rightholders on the one side and researchers and ML developers on the other”.
On the other hand, Trendacosta and Doctorow are critical. They argue that this approach will lead to market concentration and exploitation of creators by big companies. Because creative labor markets are already heavily concentrated and dominant companies have significant bargaining power, they will be able to impose contractual terms on artists that require them to sign away their “training rights” for reduced compensation. The medium to long term result would be more concentration of power with large companies leaving less control and remuneration for artists.
Whether one agrees or disagrees, the truth is that the opt-out approach is in the law and seems to be gathering momentum, both in practice and in EU policy making.
Still, there has always been a clear shortcoming in the opt-out approach of Article 4 CDSM Directive. It relies significantly on the public availability of training datasets (e.g. like LAOIN’s) in order to effectively opt-out. In other words, you have to have some way to know that your image was or will be actually used in training. For this reason, there have been increased calls for transparency requirements regarding inter alia copyrighted works in data sets, e.g. in the Authors and Performers Call for Safeguards Around Generative AI in the European AI Act (critically reported here). One clear expression of call for this type of transparency can be found for instance in Communia’s recent recommendation:
The EU should enact a robust general transparency requirement for developers of generative AI models. Creators need to be able to understand whether their works are being used as training data and how, so that they can make an informed choice about whether to reserve the right for TDM or not.
As noted, in response to this concern, in the proposed AI Act EU lawmakers are currently considering requiring providers of generative AI systems that they “make publicly available a summary disclosing the use of training data protected under copyright law.” But the devil is in the details.
Enter the AI Act: Transparency and Content Moderation Safeguards
Before we proceed, it is important to clarify at what stage we are in the legislative procedure of the EU AI Act. The proposal for this new regulation was presented in April 2021. Following the normal co-legislative procedure, the European Parliament (EP) and the Council started to discuss their own versions of the Act, based on the Commission’s proposal. The Council adopted its common position in December 2022. On the EP side, there have been the usual discussions on multiple committees, which are close to conclusion. A compromise version was leaked recently, which will likely form the basis for a vote on 11 May. If approved, that version will constitute the EP’s position on the AI Act that will go into interinstitutional negotiations in the trilogue stage, the EU political version of a black box.
It is the EP’s leaked compromise version that contains the controversial copyright provisions. These are briefly examined below, with the caveat that the version examined was leaked and is not final. (I will not discuss below an interesting reference to copyright in the rules on limitations to transparency requirements regarding “deepfakes”, but I invite copyright nerds to read Article 52(3a) if they want to learn more.)
The Copyright – AI Act interface
The first aspect to mention regards definitions. In the leaked version, a distinction is drawn between “general purpose AI system” (GPAI) and “foundation models”. Whereas a GPAI is an AI system that can be used in and adapted to a wide range of applications for which it was not intentionally and specifically designed, a “foundation model” means an AI system model that is trained on broad data at scale, is designed for generality of output, and can be adapted to a wide range of distinctive tasks (Article 3). Importantly, “generative AI” is defined as a type of foundation model “specifically intended to be used in AI systems specifically intended to generate, with varying levels of autonomy, content such as complex text, images, audio, or video” (Article 28b).
Article 28b, paragraph 5a, then imposes two specific obligations with copyright implications on providers of generative AI models, in addition to their remaining obligations as providers of foundation models. The first obligation concerns transparency and disclosure. The second refers to safeguards and can be viewed as a content moderation obligation. Crucially, providers must ensure that these requirements are met prior to making the foundation model (including generative AI) available on the market or putting it into service.
Transparency and disclosure
The transparency requirements of providers of generative AI models are twofold. First and unrelated to copyright, they must comply with the separate transparency or information obligations outlined in Article 52(1). This provision requires that AI systems intended to interact with natural persons are designed and developed in such a way as to inform the natural person exposed to an AI system that they are interacting with an AI system in a timely, clear and intelligible manner, unless this is obvious from the circumstances and the context of use. The provision then adds a number of additional information requirements regarding this human-AI system interaction.
Second and related to copyright, providers of generative AI models shall “document and make publicly available a summary of the use of training data protected under copyright law” (Article 28b–5a). This is the provision that most clearly aims at enabling opt-out under Article 4 CDSM Directive.
I will not discuss here the important question of whether it makes sense to have special rules for copyrighted material as compared to other materials in the training data, although this is certainly a discussion that must be had. Bypassing that issue for now and focusing on the proposed text, the question that arises is what it exactly means to document the use of training data protected under copyright law, and to provide a summary thereof.
Clearly, if the goal is for generative AI providers to list all or most of the copyrighted material they are including in their training data sets in an itemized manner with clear identification of rights ownership claims, etc, then this provision is impossible to comply with. The low threshold of originality, the territorial fragmentation of copyright and its ownership, the absence of a registration requirement for works, and in general the poor state of rights ownership metadata (see e.g. here) demonstrate this impossibility.
If that is the case, then it is of paramount importance to clarify the meaning and scope of this obligation. Here, the last minute addition of this requirement shows the absence of any impact assessment of its meaning, scope and implications. In the time remaining in the legislative process, the EP – as well as the Council and Commission during trilogue – should carefully consider what type of transparency is required to enable commercial TDM opt-outs, if that is the desired policy goal.
Arguably, the type of transparency that is useful is one that allows copyright holders to access datasets in order to exercise their opt-outs. It is unclear how the present text would enable that, since it imposes a requirement that cannot be met in practice. Furthermore, generative AI providers should be incentivized to collaborate with copyright holders in this process, e.g. for the development of workable standards to make effective the reservation of rights. From that perspective, it could be useful to frame the newly proposed obligation as one of good faith or best efforts to document and provide information on how the provider deals with copyrighted training data.
Safeguards for AI-generated content moderation
In addition to the transparency provision, Article 28b, paragraph 5a, adds an obligation to “design and develop the foundation model in such a way as to ensure adequate safeguards against the generation of content in breach of Union law in line with the generally-acknowledged state of the art, and without prejudice to fundamental rights, including the freedom of expression”. In addition, providers of foundation models “shall assist the downstream providers of such AI systems in putting in place the adequate safeguards referred to in this paragraph.” Although these requirements are not specific to copyright they would seem to apply also to the moderation of outputs of generative AI systems that are copyright infringing.
It is possible that certain AI generated output infringes the rights of the creators of works used during the training of the model. Generative models are able to “memorize” content they are trained on, i.e. producing identity between output and input works. Although cases of identity are theoretically possible and have been reported, they are rare. Even in the Stability AI class action lawsuit, the complaint recognizes that “none of the Stable Diffusion output images provided in response to a particular Text Prompt is likely to be a close match for any specific image in the training data” (see para 93). As Sag puts in a recent paper, “[a]t the moment, memorization is an edge case”, although there are “particular situations in the context of text-to-image models where memorization of the training data is more likely”, which “problems are accentuated in the context of copyrightable characters… and analogous situations”. If that occurs, then there is a likelihood that the output is infringing. While this is a statistically rare occurrence, what may occur more frequently is that there is similarity between the output and one or several of the input works. Under many national laws, an output would be infringing if it is substantially similar to a pre-existing work in the training data (on copyright’s substantial similarity test in US law, see here; on the complex issue of similarity of generated output and styles, see here).
In my view, these safeguard requirements with reference to state of the art technology in the proposed AI Act are reminiscent of content moderation filtering requirements. Content moderation is a topic dealt with elsewhere in EU law by the Digital Services Act (DSA) and, for copyright protect content, in inter alia Article 17 CDSM Directive. The latter provision has given rise to a rich discussion of permissible filtering, including a Grand Chamber judgment of the CJEU in Case C-401/19 – Poland v Parliament and Council (on the topic of copyright content moderation see here, here and here). Now, as Hacker, Engel and List have argued, it is difficult to apply the DSA to generative AI models, especially because it would be challenging to qualify them as intermediary service providers – in particular hosting providers – covered by the DSA. For copyright purposes, it would also be difficult to qualify them as online content-sharing service providers covered by the CDSM Directive. Naturally, if the generative AI tool is embedded in an hosting service, one might ask if it is then not regulated by the DSA as an “online interface” of a platform, as broadly defined in Article 3(m) DSA (see also Recital 70). But that is a matter for a different blog post.
The upshot is that for the most part neither the DSA nor the CDSM Directive will have much to say about the shape of the safeguards to be developed by providers of generative AI models or downstream providers of the systems. From a copyright perspective, one possible outcome is the development of filtering tools for generative AI providers to avoid the generation of infringing outputs. Although there is not much transparency on that topic, existing tools to some extent already seem to be applying such filters to avoid copyright infringement, with varying degrees of success. The examples below instructing ChatGPT, DALL-E and Stable Diffusion to “produce an exact copy of Mickey Mouse” illustrate the point.[3]
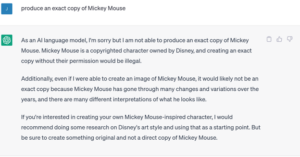
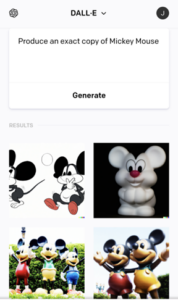
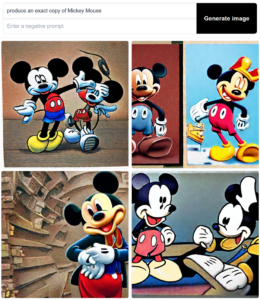
Discussion on copyright content moderation and filters under Article 17 CDSM Directive has highlighted concerns with overblocking and the need to consider the freedom of expression of users, especially as manifested in “transformative use” exceptions, like those covered by Article 17(7) CDSM Directive. To be sure, Article 28b, paragraph 5a of the leaked EP version of the AI Act makes a reference to freedom of expression. However, the fact that existing copyright acquis provisions likely do not apply directly to generative AI models may cast doubt on the need to consider them when developing filtering tools against infringing output.
As such, it would make sense in the AI Act to clarify (e.g. in supporting recitals) the need for these safeguards to fully take into account existing exceptions, namely those freedom of expression “transformative use”-type of exceptions mentioned in Article 17(7) CDSM Directive: quotation, criticism, review; and use for the purpose of caricature, parody or pastiche.
Considering the momentum behind these new provisions in the AI Act, it is expected that they will make it to the trilogue stage. Whether and how these rules will survive and be shaped during this process will be consequential to the future of copyright in the age of generative AI. Perhaps additional transparency also on this stage of the political process – not just on copyright inputs – would be beneficial for the final outcome.
Note: This blog post relies on and develops upon previous work of the author, including JP Quintais and N Diakopoulos, A Primer and FAQ on Copyright Law and Generative AI for News Media, Generative AI in the Newsroom (26 April 2023). The author wishes to thank Thomas Margoni, Martin Kretschmer, Alina Trapova and Nick Diakopoulos for comments on earlier versions of this post.
[1] On the related topic of software generation, it is also important to mention the class action lawsuit against Microsoft, GitHub, and OpenAI concerning the GitHub Copilot (reported here; case updates here).
[2] This provision may be combined with the optional exception covering uses for non-commercial scientific research purposes in Article 5(3)(a) InfoSoc Directive, which already covered certain TDM activities.
[3] Credit for the prompt goes to Professor Thomas Margoni.